Running Out of Time / Michael Donnelly
Michael Donnelly is a professional data scientist, policy researcher, and economist. He joined us to discuss epidemiological modeling of the #SARSCoV2 #COVID19 #coronavirus epidemic in the U.S. His conclusion: we’re running out of time.
You can read Michael’s Medium posts here:
https://medium.com/@donnellymjd/no-good-options-dda04260b232
https://medium.com/@donnellymjd/covid-19-nyc-should-brace-for-impact-and-shut-down-now-a7c62afac81e
This podcast was created by Just Human Productions. We’re powered and distributed by Simplecast. We’re supported, in part, by listeners like you.
Celine Gounder: I’m Dr. Celine Gounder, and this is “Epidemic.”
In this episode, I speak with Michael Donnelly, whose background is in economic research, financial policy, and data science. He’s worked in the U.S. federal government in various research positions for six years, and in the private sector for almost seven years.
Last Wednesday, Michael published an analysis to provide a broad sense of the scale of the crisis the country is facing. He found that reported cases of COVID-19 in the United States dramatically understate the current number of infected Americans. The same week, Michael published another analysis looking at the New York City Metro area. His results were startling.
The outlook for New York City and COVID-19 is bleak. The policy response has been far too slow and too weak to meet the needs of the moment. His analyses came just days before an Imperial College report predicting high fatalities in the U.S. if the country doesn’t take extreme measures.
Michael Donnelly: The place where I start with all of these conversations is that I’m not an epidemiologist, and I’m not a public health researcher. My background is more in economic research, and financial policy and data science. So, prior to my current job as a data scientist in the tech sector, I worked at the federal government for about six years, and in the private sector for about three years in financial policy research. And so my specialty in all of this is quantitative communication and data science and forecasting.
Celine Gounder: How did you decide that you wanted to delve into this? I mean, this is not your regular job, right?
Michael Donnelly: No, it really isn’t. It was really, it was a fascination, I think is, to be totally honest, it’s kind of the topic that I’ve always been interested in.
I did some training in health economics in grad school, and I started digging into this really in January, and was pretty convinced by February that this was pretty major, even for the U.S. I found over those weeks that I was getting pretty exhausted trying to convince friends that this was not the seasonal flu.
Um, and so because of my background, my skill set, I turned towards quantitative analysis and empirical research to answer these questions first. And that’s, you know, that’s really where I started going, um, to begin with. So really in March, after becoming pretty tired of trying to convince friends, I decided it’d be a lot easier to compile all of my thoughts in written form.
And so like, and also providing that really is a resource for people to feel empowered to explore the existing scientific research on the topic, um, rather than just believing some friend of theirs who thinks he’s got a good opinion. Um, so I started writing up, about a week and a half ago, um, all of my notes, and it quickly turned into like a 20 page, um, Medium post with, uh, the research and my own quantitative analysis and modeling.
And before I put it out there, because it had some disturbing conclusions to it, um, I ran this by a bunch of friends. I think probably about six to eight of my friends who I had review it had PhDs, JDs, or some sort of doctorate in a variety of fields between, um, public health, economics and political science and law.
So I was, I was really making an effort not to put out that information, but my main goal was to put out information at a level that you didn’t have to have a degree in science or in statistics to understand. Um, so that was my goal with my first Medium article. It was, it was really kind of a national overview.
Shortly after that, I think I released it a week ago today, I started thinking through the consequences of my own life. And part of that meant thinking about, um, my rec sports league, which was about to restart its season. And so, I ran, as I realized this, the executive board meeting was starting in about a half an hour, and so I ran down to the, to the board meeting to ask them to cancel the upcoming season. And as I was waiting for my turn to speak, I replicated my national analysis, uh, for the New York City region, and, uh, got pretty disturbing results about what that meant for New York’s healthcare system and its capacity to absorb, uh, you know, the forecasted severe and critical cases into hospitals.
That was the realization that I needed to do a more formal analysis for New York, and by the next day around lunch, I had finished the analysis and was ready to post it on Twitter after getting some other friends to review that specific analysis as well. That’s when I started reaching out to all the government officials that I could possibly reach out to because the conclusions were so dry.
It really told me that we had very little time left to take much, much stronger governmental action to increase social distancing before we had essentially locked in too many infections that would overwhelm New York’s hospital system.
Celine Gounder: Some follow-up questions about that. So, having looked at sort of the national scenario, as well as the New York City scenario, how divergent or different are those?
Michael Donnelly: Well, I’ll start off by saying that there are a lot of models out there that do a really good job dealing with, uh, different geographies and densities and the way that people move around in different ways based on different cities. Those models also are unfortunately very complex and difficult to understand.
The models that I used are, essentially, a simple, almost a hundred-year-old models called FIR models, and they just work at the top line, summed up the whole country or summed up the whole city. Because they have the same structural form, if you are saying that the virus progresses in the same way, they kind of look very similar, in terms of how quickly they progress under these simple models.
So that’s kind of the short answer. The wrinkle in that is that it matters a great deal about where you estimate your current position to be, um, versus the relative total susceptible population. So, in the New York City region, it really seems that, while I haven’t done a formal analysis of all the cities in the U.S., it seems like it’s the most, the highest priority city to be addressing the problem right now. Um, you could also think that maybe Seattle would be close on its heels.
Celine Gounder: To some people, that would be surprising because Seattle was hit first. Why would that be?
Michael Donnelly: I’ll try and point out throughout this conversation where I’ve done what I think is firsthand quantitative analysis, and where I feel like I’ve got greater uncertainty. In this place I haven’t done as much firsthand quantitative comparisons, but I think, um, it’s important to point out that it seems that most of Seattle’s cases, at least detected cases up front, were in a nursing home among a much more susceptible population, a much more vulnerable population, among older people.
Whereas in New York, you had its first cases detected among 50 somethings, 40 somethings, who are very mobile throughout the city and didn’t have a clear lineage to trace back how they had become infected in the first place. And so, since it could really have been, you know, if you know New York City, anywhere along the Metro North Corridor or in the subways of Manhattan, it stands to reason that there were substantial numbers of undetected cases that were circulating around America’s most dense city.
And so, for that reason, I think it’s reasonable to assume that the overall reproductive rates that had been detected in a variety of studies based either on the experience of China, or the experience on the Diamond Princess Cruise, which those studies I leverage heavily my papers because they really could do a full population study, uh, in, in those papers. I think they give you a really good picture of what to expect in New York without intervention.
Celine Gounder: How, um, are you able to guesstimate, estimate, you know, how far back coronavirus may have been introduced into New York City when, when the first cases may have started circulating here?
Michael Donnelly: Yeah, I didn’t do that analysis, um, but the last time I updated the analysis was the end of, uh, March 13th, which was last Friday. As of that time, we had about 400 cases in the New York City region that were reported, and we were estimating anywhere from 1300 to 2300 cases overall in the regional population, but most of which hadn’t been detected.
That suggests that it had been in New York City for many weeks, but you know, I mean, greater specificity than that, I couldn’t, I’d just be guessing.
Celine Gounder: So maybe if you can just talk us through, first of all, you know, what you found. You sort of gave us, um, a very, very broad overview of what that was, but maybe a little bit more specifically.
Michael Donnelly: Sure. The numbers that I found in New York City, which are now five days old, about a third of the analysis is just focused on trying to understand the actual current number in the population of infected and potentially infectious cases. As I mentioned before, we had reported at the time, 400 cases, and then there’s a real question of, okay, how do you kind of ballpark the right number of cases in a population. You know, the place to start is to just acknowledge that directionally, the reported number of cases is an underestimate, assuming also a fairly low false positive rate in the testing results. Although that, that may be somewhat of a weak assumption, given some reporting that I’ve seen on that.
But regardless, even with a relatively high false positive rate in testing, it’s almost certainly an underestimate because of the limited number of tests out there and the strong restrictions that even still hold to this day about who can be tested. But there were even stronger restrictions on who could be tested at the time last Friday.
So, the approach for coming up with the estimate, which I detailed a lot more in my first paper, was to attempt to estimate four different parameters, and those parameters were the asymptomatic rate, mild case rate, and then two detection rates. So, I’ll start first on the why we want to look at the asymptomatic and the mild case rate.
Mild and asymptomatic cases are just a lot harder to catch because they present, as you know, as a kind of a cold, and people don’t get tested for colds regularly. And so, we kind of need to know first how many people who get COVID-19 are going to experience these mild new symptomatic versions of it. So, I went to a few different papers.
There’s a couple of papers on the clinical characteristics of the virus coming out of China, one in The Lancet that I used and one in the New England Journal of Medicine, and they both place the mild case rate at around 80%. I think one had 81%. And both papers, uh, had about a 1% asymptomatic rate, if I’m remembering correctly.
Whereas there were two papers coming out of the Diamond Princess Cruise, which was, unfortunately for the passengers, a pretty good natural experiment for research purposes because you can test everyone on the cruise. They came up with a couple of different estimates for the rate of asymptomatic cases. One paper placed that at the lower end, around 17%, and another paper placed it at the higher end, around 35%.
So why does that matter? If we believe both papers, then the easiest way to reconcile them is to say that the clinical papers coming out of China just weren’t getting into their sample, the asymptomatic cases, because those aren’t labs confirmed cases. And it makes sense that they would be missing quite a few asymptomatic cases based on what I know about the Chinese testing protocol.
So, how to adjust then this mild case rate, it’s actually not that hard. You just assume that if the overall population actually has a 35%, um, asymptomatic cases. Then of the remaining 65%, 80% are mild. So then you can just add up those percentages and you can get the overall mild and asymptomatic rate. And it’s both mild and asymptomatic patients tend to be harder to detect. We then separate out the detection rates for these non-severe cases, and for severe and critical cases. By the way, the calculation of the severe and critical case rate is much easier. Once you’ve figured out the mild and asymptomatic rate, you can just subtract that from one, and you get the remainder is going to be severe and critical cases, which ends up being somewhere just under 10%, depending on which estimates you use.
The next step, once we’ve kind of got that, is to just understand how quickly people transition from one population to the other.
Celine Gounder: So there’s also been a lot of discussion, as you know, about, um, school closures, mass gatherings. Those are two slightly different questions. Based on your modeling and your looking at the evidence, and I think you’ve been, uh, involved in the discussions, at least in New York City, around school closures. What do you think the evidence shows and what’s been your involvement in those discussions?
Michael Donnelly: It does seem to show in various studies that I’ve been reading that, you know, approximately a third of transmissions happen, uh, at schools and at workplaces. And so closing down schools, while children don’t seem to have as many severe side effects from this, they do seem to be a vector. And there was one interesting analysis that I saw coming out of South Korea where they’ve done really extensive testing of their population, and a lot of people have pointed to that extensive testing as being one of the reasons they’ve been able to get the spread of the virus under control there.
But the finding was that there was a quote unquote “over indexing” of cases among 20 to 29-year-olds. And so even though they don’t tend to show as many symptoms or as many severe symptoms, it seems that because it’s a more social group in the age pool that they’re one of the biggest vectors for the disease spreading.
And so, while that’s not the age group for public schools, it is a part of the age group for universities. I think between the Imperial College study that found that a significant part of transmission happens in schools, and the finding coming out of South Korea that a significant part of the transmissions are coming from 20-somethings, it does make sense that we’re going to want to continue doing, uh, restrictions on movements and restrictions on schools and universities.
Celine Gounder: How different are your methodologies? I mean, it sounds like some of it may be that you’re just trying to approach it as simply as possible, especially for lay people to be able to understand what you’re doing.
But how different are your methodologies from, say, Ferguson, Neil Ferguson or Marc Lipsitch?
Michael Donnelly: The Ferguson paper, this is the Imperial College paper, uses what I believe is an agent-based model. And so this effectively means that they’re attempting to model the individuals and society and how they move about, and how much they contact each other. This is a pretty new approach to modeling, at least in my background in financial stability research, and it can yield some interesting results that are different than what, um, top line statistical analyses yield. But on average, it turns out that they yield very similar results. And so, what I can point to is the conclusions that both Neil Ferguson and I reach and what parameters we use to reach them. The Neil Ferguson paper uses an R0 of 2.4, which is almost the same as my 2.3. They, I think, use essentially a serial interval, so this is how frequently that compounds, of just over five days, which is slightly more than my 4.7.
They find for the U.S. that, without intervention, about 80% of the U.S. population would become infected. I find higher than that, but, um, it would make sense that there’s slightly different results, particularly on that factor.
And then finally, the total number of deaths, which is a super important metric, I think we would all agree. They find, uh, about 2.2 million deaths peaking sometime this summer, um, which is slightly later than my timing estimates of that. But it’s also just under twice as high as my estimate for that. From a forecasting and statistics standpoint, all of these results are, you know, what we’ve kind of referred to as within an order of magnitude.
So while they approach the modeling from a pretty different mathematical standpoint, what’s interesting is that the results come out very similarly. And when you’re using models to forecast, it’s actually a good thing to have people pursuing a lot of different model forms, because if they’re all coming out with roughly similar conclusions, um, and they’re not collaborating, then you can have a much higher degree of confidence in the findings.
Celine Gounder: So in other words, you’re approaching the problem in different ways, but you’re all sort of, your results are all pointing in the same direction.
Michael Donnelly: Yeah, that’s exactly right. And part of the reason I chose a simpler model, aside from the fact that I’m not an epidemiologist, was also, I think it’s important for people to feel like they have some agency over understanding why political leaders are taking such strong actions when it seems like the death rate, or the critical case rate, is still pretty low in the country. And so I hope that this math, and this explanation of the math, gives people a better sense of why it’s important to act now.
Celine Gounder: You know, another thing that’s been up for debate is duration of the social distancing measures and when we can lift them. What does your model show and how does that align with the, um, Ferguson model?
Michael Donnelly: I haven’t done what the Ferguson model did. My analysis went up through the first part of the Ferguson model, and it aligns very well with their hypothetical no government intervention and no individual response to the, to the virus. Our results are within essentially an order of magnitude of each other and have very similar peaks.
In terms of the interventions, I think that there’s limited information right now to estimate exactly how big an effect each of these interventions have on the virus. And so, the Ferguson approach, using an agent-based model, is some of the best ways that we can deal with that right now. I do think that there’s going to be more evidence coming out of the change in the reproductive rate in each of the countries that we’ve seen- China, South Korea, Japan, France, Spain, Italy, to see how much we can attribute change in reproductive rates to these policy implementation. There’s just very limited data on that right now, so I haven’t attempted that work yet. One of the things that we’re beginning to discuss amongst some other U.S.-based data scientists is trying to come up with a way of tracking, at least directionally, in real-time changes in the reproductive factor in different regions of the United States.
But in order to do that we’re also going to have to estimate changes to the detection rates, and that’s all related to the number of tests as a proportion of the number of infections. So in order to do that, we’re going to have to continue to forecast. So, there’s a lot of unknowns right now, and it’s a big mathematical problem essentially, and a data problem that a lot of people have just begun to work on.
So I’m hopeful to have a better answer for that in the coming weeks, but right now I think that the Ferguson paper is one of the best ones I’ve seen on it, and they seem to suggest that the first period of lockdowns is going to be the longest because we need to get the disease spread under control before we can think about lifting the lockdowns, so that we don’t overwhelm hospitals immediately after lifting them. And I think they place that somewhere around two months for the initial period.
Celine Gounder: Then, you lift the measures, but then you have these subsequent waves of disease. So how do you decide when to reimpose measures and can you do so in a more local fashion as opposed to doing so across the country?
Michael Donnelly: I think there’s a couple of points there. One key point to get first, to understand the intuition behind the subsequent point, is that ICU-cases, or severe hospitalized cases of coronavirus, are a lagging indicator. So, if you stop people from catching the virus by imposing a lockdown when you’re at 100% of your hospital capacity, well you’ve got all the transmission that had been happening a week before then that haven’t been showing the symptoms. And so, you’re going to have all of those cases that haven’t shown up at the hospital yet, are arriving at your hospital after your hospital is already full over the next week. So, what the paper does is it says, we’re going to need to estimate capacity triggers for this. And I think that there’s a real data problem here.
So let’s say that the capacity trigger is like a third of the, of the available capacity in the hospital, and that seems kind of reasonable to me. But in order for that trigger to be in place, we’re going to need to know what the capacity of the hospitals are in the region, and what the number of hospitalized cases are in the region.
And as far as I can tell, and I have been speaking to epidemiologists and data scientists across the country, there doesn’t seem to be a good centralized source for that information. And so, it’s going to be a lot harder for even sophisticated state and local governments to do that. And I think as unsexy a problem as it is to say, we need to invest in data right now, I think that’s gotta be a high priority.
Celine Gounder: How well do both models predict how things have played out in Italy and how things have already started to progress here in the U.S.?
Michael Donnelly: You know I haven’t seen many back tests of the Italy case. I can only speak to my own on that one, and when I ran it on about two-week-old data coming out of Italy, my model, it was actually somewhat underpredicting the two week out number in Italy. Now, that is essentially within what we expect to be the error for a model, and so it’s suggested that these models, and thus the parameters that we use for the models, that the are not that reproductive rate, how frequently that compounds, those sorts of things. It really suggests that these models are right and left broadly unchecked, like they were in Italy, the future extrapolations, even out a month or two, are right. And I think that’s really why people, why governments are beginning to take this so seriously right now because people have really convinced, uh, national leaders that if they don’t do something now, these really horrific scenarios could play out.
Celine Gounder: How far out can you accurately forecast and how, you know, how, how much can we trust these predictions?
You know, some people are saying three months, five months of these kinds of interventions, you know, how long, um, are we looking at this? You know, are we going to have to really wait 18 months for a vaccine? You know, what can you say about the, um, how far out you can really project?
Michael Donnelly: I think with almost any forecast, the underlying fact is that the further out you try and forecast, the less accurate you’re going to be.
So, a year from now is always going to be harder than a month from now. And a month from is always going to be harder than a week from now. In this case in particular, uh, the biggest source of uncertainty, at least from my perspective, is the policy response. The models that I’ve done and the models I’ve compared my work to, particularly the Imperial College model done by Neil Ferguson and his team, they’ve been criticized in what I think is a productive way. For one, for instance, they haven’t considered the case tracing that could happen alongside some of these other policy implications, and that in fact could reduce, uh, future infection rates. Similarly, in their paper, they suggest doing essentially on and off, and if locked down or suppression tactics, um, in order to deal with both the economic and the health policy consequences of these, uh, policies of keeping people indoors and, and social distancing. And that would essentially flatten the curve by having the curb kind of go into waves.
But those waves over time may not be evenly spaced, and those waves may not be evenly spaced for a variety of reasons. One is increasing technology, increasing test loads, but also increasing, um, acquired immunity because more people have been exposed. So, those sort of dynamics are very challenging to forecast, and I think what a lot of people who are involved in trying to forecast this right now are doing, is they’re trying to do, you know, two steps forward, one step back, and I think we’re just going to keep it leveling up the quality of these forecasts over time as we decide which forecasting approach, um, is yielding the best results.
Celine Gounder: Do you think, um, New York City has acted quickly enough, and for that matter, the U.S.?
Michael Donnelly: Uh, the short answer is no. I think we knew enough last week to have taken the action that the mayor took on Sunday, which is to close restaurants, bars, public venues, movie theaters, and schools. Now schools, it doesn’t matter as much over the weekend, but bars, clubs, I think they were reduced to quote unquote, 50% capacity, I believe, by the governor. But I don’t believe that most of those venues were observing that order. At some point, I think, uh, some clever researchers are going to figure out just how many illnesses are to blame for that slow movement. And, I know more about the New York situation than the national one, but I think New York has some of the best health surveillance systems in the country, and I think that’s probably why we know more about New York. We also probably have more because it’s a more dense city. But, I could see a similar pattern playing out across the country, and it certainly hasn’t helped anything when you have national leaders telling people that it’s a hoax, and that it’s not even as bad as the seasonal flu. People will take action to protect themselves, but they won’t do it if they don’t think it’s real. Even just those words, um, have been a detriment to the spread of the virus in the country, and those sorts of things are going to cost people lives.
Celine Gounder: Yeah. I mean, I can’t agree with you more. How, how soon do you think we might see hospitals in New York overwhelmed by this?
Michael Donnelly: That’s a tough question. It’s hard to know how much the decision to close bars and restaurants and other public venues over the last couple of days has slowed down the virus. The model that I published on March 14th using data through March 13th suggested that we were going to hit enough infection by March 23rd or 25th, that by the beginning of April we would be at hospital capacity. Some recent analysis that I’ve done using data from yesterday and today suggests that I was undercounting the starting place in the New York City region.
Um, the trend was accurate, but it’s generally bad news. I’m very nervous for the hospitals in New York City. I think the news that the, uh, the defense department sent the hospital ship to New York City, which is frankly unimaginable a month ago, is actually very good news and will hopefully help with the surge capacity, but from what I hear, the outer boroughs of New York have only seen a 50% reduction in subway travel when you look at the, uh, the turnstile data that comes out of the MTA. I don’t think 50% reduction is enough if we are already at capacity in the number of infections we have today, which we may be at. So, we may be adding beyond capacity every day that goes by that New York doesn’t adopt stronger lockdown procedures.
Celine Gounder: Michael, before we close, is there anything else you wanted to make sure to get across or wanted to add?
Michael Donnelly: I’m impressed by people’s desire to get involved and from a wide variety of walks of life. You know, I think there’s a lot of volunteer work that’s coming out. I think we’re resilient. I’ve seen people who work in gyms, who are personal trainers, who lost their income source for a long time, immediately hop online and find ways of making income for themselves by doing video fitness classes. I think we’re just going to have to be creative going forward to get through this. And honestly, I think there’s some opportunities for really good things to come out of this. It’s going to be painful in the meantime, but I’m not fully pessimistic about the next few months.
Celine Gounder: “Epidemic” is brought to you by Just Human Productions. Today’s episode was produced by Jordan Gasporay and me. Our music is by the Blue Dot Sessions. If you enjoy the show, please tell a friend about it today, and if you haven’t already done so, leave us a review on Apple podcasts. It helps more people find out about the show.
You can learn more about this podcast, how to engage with us on social media, and how to support the podcast at epidemic.fm. That’s epidemic.fm. Just Human Productions is a 501(c)(3) nonprofit organization, so your donations to support our podcasts are tax-deductible. Go to epidemic.fm to make a donation.
Starting next week, we’ll be releasing “Epidemic” twice a week on Tuesdays and Fridays, but producing a podcast costs money. We’ve got to pay our sound engineers, so please make a donation to help us keep this going.
Also, check out our sister podcast, “American Diagnosis.” You can find it wherever you listen to podcasts or at americandiagnosis.fm. On “American Diagnosis,” we cover some of the biggest public health challenges affecting the nation today. In season one, we covered youth and mental health, in season two, the opioid overdose crisis. And in season three, gun violence in America.
I’m Dr. Celine Gounder.
This is “Epidemic.”
Guests
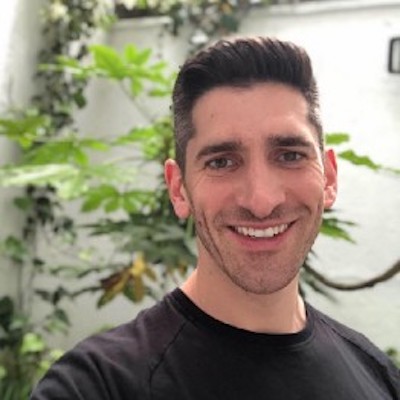